Introduction to Biotech’s Technological Evolution
The biotech industry has witnessed a remarkable transformation in recent years due to rapid technological advancements. Among the most revolutionary fields shaping biotechnology are artificial intelligence (AI) and machine learning (ML). These cutting-edge technologies have opened new avenues for researchers, scientists, and healthcare professionals, offering unprecedented insights and efficiency in biotech research and development.
The convergence of AI and biotech holds immense promise for accelerating drug discovery, personalizing medicine, optimizing bioprocessing, and advancing diagnostics. This article delves into emerging technologies in biotech, focusing on the role of AI and machine learning. We will explore real-world examples, potential applications, ethical considerations, and the prospects of these technologies in revolutionizing the biotech landscape.
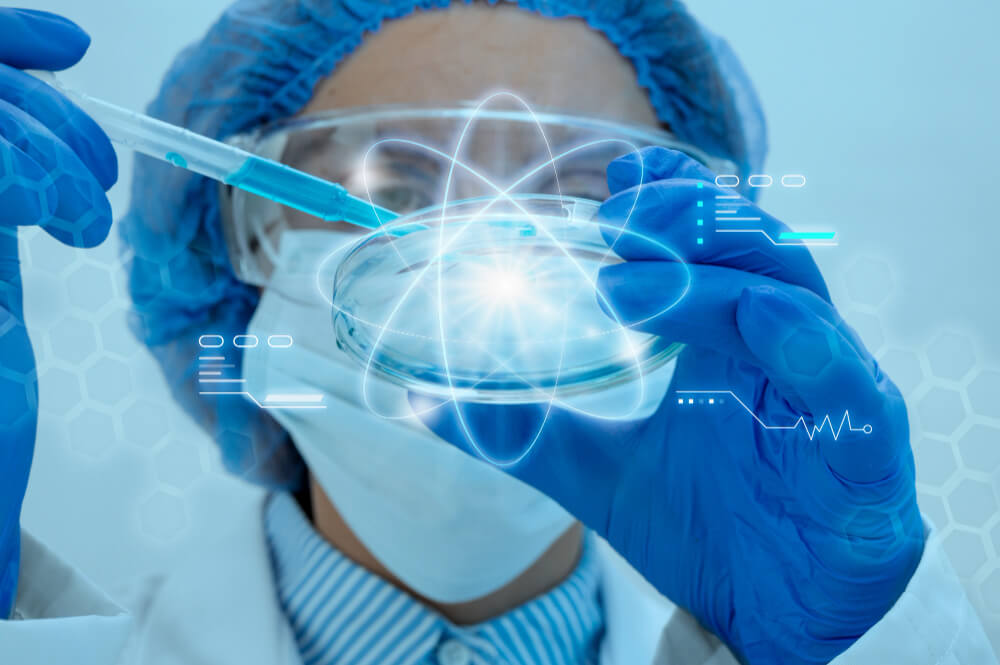
The Synergy of AI and Biotech
AI and biotech are two fields that, when combined, form a powerful synergy. In various forms, AI provides algorithms and computational models that can analyze vast datasets, recognize patterns, and make predictions. Biotechnology, on the other hand, deals with biological systems, genetic information, and the development of therapeutic solutions.
Integrating AI in biotech research has resulted in groundbreaking discoveries and significantly accelerated progress. For instance, AI-powered algorithms can process and analyze massive genomic datasets that would take years for traditional methods. This enables researchers to uncover genetic variations, identify disease biomarkers, and understand complex genetic interactions more efficiently.
Real-world Examples of AI Applications in Biotech
Drug Discovery: AI is transforming the drug discovery process by automating high-throughput screening of chemical compounds and predicting their potential efficacy against specific diseases. This approach expedites the identification of drug candidates, reducing the time and cost required to develop new treatments.
Genomics and Personalized Medicine: AI-driven genomics research has led to a deeper understanding of individual genetic variations and their impact on health and disease. ML algorithms can predict disease risks and recommend personalized treatment plans based on an individual’s genetic makeup, leading to more effective and targeted therapies.
Precision Agriculture: Biotechnology and AI reshape agriculture by optimizing crop breeding and cultivation. AI-powered systems analyze environmental data, plant genomes, and agricultural practices to enhance crop yields, conserve resources, and develop disease-resistant varieties.
Machine Learning in Genomic Research
Genomics, the study of an organism’s complete set of DNA, presents a vast landscape of data. Extracting meaningful insights from genomic data is challenging and essential for advancing personalized medicine and understanding genetic diseases. With its ability to detect patterns and relationships in complex datasets, machine learning has emerged as a critical tool in genomic research.
Leveraging ML for Genetic Variation Analysis
One of the primary applications of ML in genomics is analyzing genetic variations, including single-nucleotide polymorphisms (SNPs) and structural variations. ML algorithms can identify patterns associated with specific diseases or traits, aiding in discovering potential therapeutic targets.
Predictive Models for Disease Risk Assessment
Machine learning models can be trained on large-scale genomic datasets from diverse populations to predict an individual’s risk of developing certain diseases. These predictive models offer valuable insights for early intervention and preventive healthcare strategies.
Accelerating Precision Medicine
In precision medicine, treatment decisions are tailored to an individual’s genetic profile and health status. ML algorithms are pivotal in predicting a patient’s response to specific medications and treatments, enabling personalized and more effective therapies.
AI-Driven Drug Discovery
Traditional drug discovery is time-consuming and costly, often taking years to identify potential drug candidates. The integration of AI in drug discovery has revolutionized how researchers approach this crucial aspect of biotech.
Target Identification and Validation
Identifying suitable drug targets is the first step in the drug discovery process. AI models analyze biological data, such as gene expression profiles and protein structures, to pinpoint potential targets involved in disease pathways. This significantly accelerates the target identification phase.
Lead Optimization and Virtual Screening
AI algorithms optimize drug leads by predicting their binding affinity to target proteins and evaluating their pharmacokinetic properties. Additionally, virtual AI screening allows researchers to sift through vast chemical databases to identify promising compounds for further testing.
Accelerating the Drug Development Pipeline
By reducing the time and cost required for various stages of drug discovery, AI-driven approaches can accelerate the drug development pipeline. This opens new possibilities for developing treatments for rare diseases and previously considered economically unviable conditions.
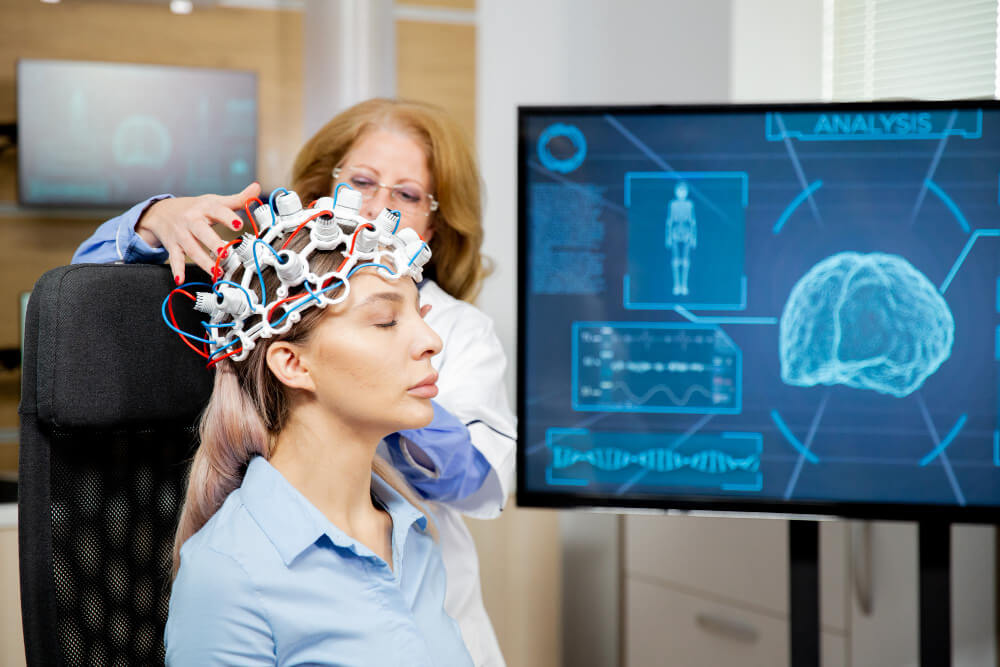
Enhancing Diagnostics and Disease Detection
Accurate and timely diagnosis is critical for effective disease management and treatment. AI and machine learning have shown great promise in improving the accuracy and efficiency of diagnostics across various medical fields.
Medical Imaging Analysis
AI-powered image recognition and analysis tools have demonstrated exceptional performance in interpreting medical images, such as X-rays, MRIs, and CT scans. These tools assist radiologists in detecting abnormalities and providing faster and more accurate diagnoses.
Pathology Data Analysis
Pathologists deal with vast amounts of complex data in diagnosing diseases. ML algorithms can analyze histopathology slides, aiding pathologists in identifying cancerous cells and other pathological features more accurately.
Predictive Diagnostics and Risk Stratification
Machine learning models can analyze patient health records and identify patterns associated with specific diseases. This enables clinicians to predict disease risks and stratify patients based on their likelihood of developing certain conditions, leading to proactive and personalized healthcare interventions.
AI-Assisted Bioprocessing and Biomanufacturing
Bioprocessing and biomanufacturing are critical aspects of the biotech industry, involving the production of biopharmaceuticals, vaccines, and other biological products. AI technologies optimize these processes, enhance efficiency, and ensure product quality.
Process Optimization and Quality Control
AI-driven process optimization utilizes real-time data monitoring and analytics to fine-tune bioprocessing parameters, ensuring higher product yields and consistency. AI models can also predict potential deviations in the manufacturing process, allowing proactive quality control measures.
Continuous Biomanufacturing
Traditional batch-based biomanufacturing can be resource-intensive and time-consuming. AI enables the transition towards continuous biomanufacturing, where production is streamlined and quality control is integrated throughout the process.
Drug Formulation and Delivery
AI algorithms assist in optimizing drug formulation and delivery systems. By analyzing drug properties and patient data, AI can recommend personalized dosage forms, routes of administration, and drug delivery devices.
Ethical and Regulatory Considerations
As AI and machine learning become increasingly integrated into biotech research and healthcare, addressing ethical considerations and regulatory challenges is crucial to ensure responsible use and patient safety.
Data Privacy and Security
AI in biotech relies heavily on vast amounts of patient data. Ensuring the privacy and security of this sensitive information is paramount, requiring robust data protection measures and compliance with data regulations.
Algorithm Bias and Fairness
AI algorithms are only as good as the data they are trained on. Biases present in training data can lead to biased outcomes, impacting patient care and treatment decisions. Efforts must be made to develop fair and unbiased AI models.
Regulatory Approval and Compliance
Regulatory bodies are critical in evaluating and approving AI-driven medical devices and treatments. Appropriate guidelines and frameworks for assessing AI-based technologies are essential for widespread adoption.
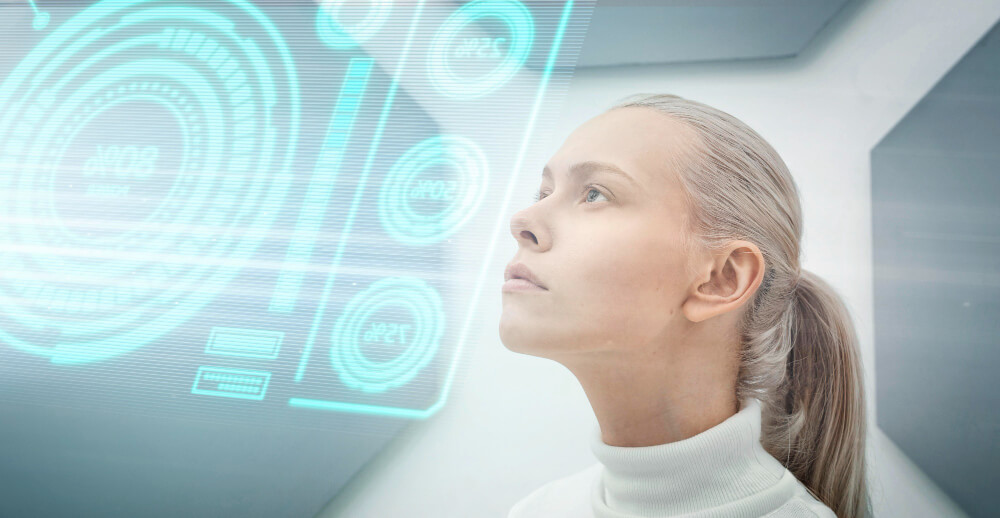
Future Prospects and Challenges
The future of AI and machine learning in biotech is promising, with ongoing research and innovation fueling new applications and discoveries. However, several challenges must be overcome to harness these technologies’ potential fully.
Interdisciplinary Collaboration
AI and biotech are complex fields that require collaboration among researchers, data scientists, clinicians, and industry experts. Bridging the gap between these disciplines is essential for driving innovation and translating research into practical solutions.
Data Standardization and Sharing
Data standardization and open sharing are crucial for training robust AI models and fostering scientific advancements. Encouraging data-sharing initiatives and establishing standard data formats will facilitate collaborative research.
Regulatory Adaptation
The rapid pace of technological advancement often outpaces regulatory frameworks. To ensure the responsible and safe deployment of AI in biotech, regulators must adapt swiftly to address emerging challenges and risks.
Overcoming Technical Limitations
AI technologies, while powerful, still face technical limitations. Addressing issues such as interpretability, explainability, and model robustness remains a priority in building trust in AI-driven biotech applications.
Embracing the AI-Driven Biotech Revolution
Integrating AI and machine learning in biotech has ignited a transformative revolution, propelling research, diagnosis, and treatment to unprecedented heights. As we venture further into the future, stakeholders in the biotech industry need to embrace these emerging technologies responsibly, addressing ethical concerns, promoting interdisciplinary collaboration, and navigating regulatory challenges. By doing so, we can unlock the full potential of AI in biotech, shaping a healthier and more innovative world for generations to come.